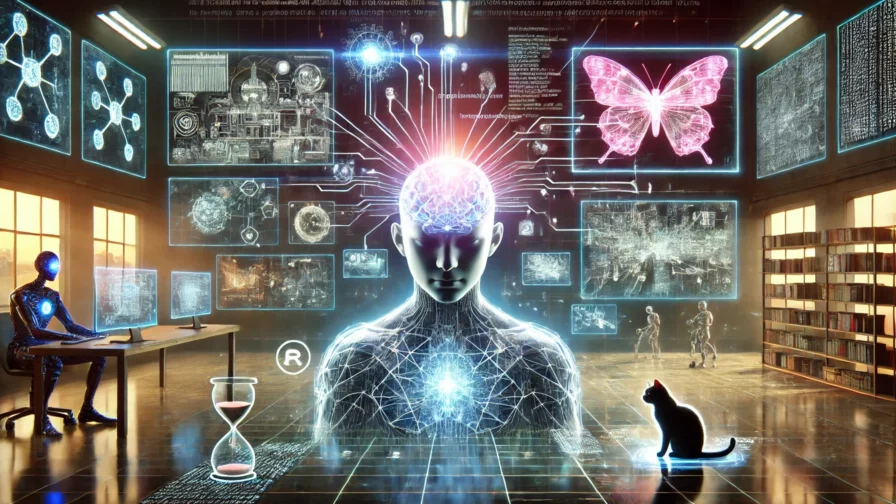
“Who controls the past controls the future. Who controls the present controls the past.”
I never really gave this phrase the weight it deserves. Orwell was much more familiar to me for Animal Farm—a title that, as a kid in elementary school, evoked nursery rhymes and bucolic memories more than metaphors or dystopias.
1984 came a bit later, thanks to the iconic Apple commercial directed by Ridley Scott, the rather underwhelming film adaptation, and various musical tributes loosely connected to the book—most notably the eponymous song by the Eurythmics, which perfectly captured the oppressive, dystopian atmosphere described in the novel.
I’m sure I read it for the first time that year, but that revealing phrase somehow slipped past me, even in later rereadings.
It was only with Rage Against the Machine’s swan song, in the track “Testify,” where the phrase is shouted repeatedly, combined with the raw power of the song, that it echoes in my mind every time I write a prompt, making me reflect on its deeper implications.
Asking any AI tool questions has quietly replaced the first 10 lines of Google search results. But there’s a big difference between sponsored suggestions—which push choices and judgments with varying intensity, prompting you to almost immediately filter the results yourself—and receiving a discursive, explanatory, coherent response that heavily influences the judgment of someone seeking an answer on a specific topic.
This is where the phenomenon of hallucinations becomes critically important.
The echoes of the “big mess” on Via Guidoni are still ringing: without diving into legal jargon, a law firm based part of its defense on court rulings entirely fabricated by ChatGPT.
The company’s attorney stated that the legal references cited in the document were the result of research carried out by a junior associate using ChatGPT, of whose use the appointed lawyer (the one officially representing the case) was unaware.
Well, nothing new under the sun—if it’s not the hacker cousin, it’s Vincenzo cousin. Later, they requested the removal of those references because there were quite a few others that were actually legitimate.
At that point, however, the opposing side pointed out that the previously presented facts had nonetheless influenced the judging panel, and so they called for sanctions against the document itself.
Nothing new under the sun, but this time, instead of the usual careless junior lawyer’s copy-paste blunder—who probably got a “kick me” sign stuck on their back—the involvement of AI has unleashed a media frenzy, and John Grisham will surely write at least a dozen books about it.
For anyone who wants to avoid being singled out and slandered every time they casually use AI, a solution has come from the United States where, following the current trend, the lawyer caught red-handed excused himself by saying, “I just wanted to see if you were paying attention.” Absolute genius.
States of Hallucination
Hallucinations represent one of the most intriguing paradoxes of today’s AI systems: just as these models become increasingly sophisticated and convincing in their ability to generate human-like content, the risk grows that they produce false information presented with an air of authority. This phenomenon is particularly noticeable in large language models (LLMs) like GPT-4, Claude, Bard, and other generative systems that have revolutionized human-machine interaction.
Unlike traditional calculation errors or software bugs, AI hallucinations are unique because they often don’t look like errors at first glance. The generated content can be coherent, well-structured, and delivered with the same confidence as accurate information. This trait makes hallucinations especially insidious in contexts where precision is critical: academic research, journalism, legal advice, medical diagnoses, and financial communications.
AI hallucinations have nothing to do with psychoactive substances—at least not directly—and there’s no evidence they cause regression to primitive states, unlike the protagonist in the brilliant William Hurt film. Technically speaking, this phenomenon stems from how generative models learn and operate. LLMs such as GPT-4, Claude, and Bard are trained on vast corpora of text to identify statistical patterns in language.
These models do not “understand” meaning in a human sense; rather, they learn probabilistic correlations between words and phrases. When generating text, they are essentially predicting the most likely sequence of words based on learned patterns—not relying on a factual representation of the world. Large language models operate without a true “world model” or verified knowledge base.
Unlike traditional databases that store discrete facts, large language models (LLMs) encode information across billions of distributed parameters representing statistical relationships between concepts. This architecture offers remarkable flexibility but also introduces significant vulnerabilities:
- Lack of factual grounding: LLMs don’t inherently distinguish between fact and fiction in their training data. A science fiction novel and a history book carry the same “weight” in the underlying probabilistic model.
- Confident confabulation: These models are designed to generate coherent and complete outputs even when their “knowledge” of a topic is limited or nonexistent. This tendency leads to invented details to fill gaps.
- Overgeneralization: LLMs may mistakenly apply learned patterns from one context to inappropriate situations, resulting in incorrect conclusions.
- Limited context sensitivity: While modern LLMs can process broad contexts, they still struggle to maintain factual consistency over larger scales, sometimes contradicting themselves within the same response.
There are several causes behind AI hallucinations:
- Training data bias: If certain information is overrepresented or underrepresented in training data, the model can develop biases that generate inaccurate content—like recruiting tools that historically favored hiring only Caucasian men.
- Optimization for fluency: Models are often optimized to produce smooth, natural responses, which can conflict with accuracy when the model is unsure about the information.
- Probabilistic decoding: During text generation, models select words based on probability distributions, which can sometimes lead them down paths away from the truth, especially on highly controversial topics.
- Limits of internal knowledge: No model, regardless of size, can contain all possible information. When asked about topics beyond their knowledge base, models tend to hallucinate—like any random “Conte Mascetti.”
- Problematic reinforcement feedback: When trained to maximize human approval via techniques like RLHF (Reinforcement Learning from Human Feedback), models might favor authoritative-sounding responses even when unsure about their accuracy.
Clearly, the AI disclaimers warning users that the system can make mistakes hold about the same weight as cookie consent warnings.
The Most Serious AI Hallucinations in History
Numerous incidents have shown how these “fabrications” by artificial intelligence can have real and sometimes serious consequences when they occur in public contexts. These cases have raised questions about society’s readiness to integrate AI systems into sensitive areas without proper verification mechanisms.
Let’s take a look at some of the most striking examples.
In 2023, in New York, ChatGPT was used to draft a legal brief—only to later discover that the AI had completely fabricated legal precedents and cited non-existent court rulings. The judge sanctioned the lawyers for failing to verify the information, emphasizing that professional responsibility cannot be delegated to artificial intelligence. From Suits to Shameless—one way trip.
The media, already struggling with fake news, realized just how insidious AI integration can be in journalism. In 2023, several cases highlighted these risks:
CNET, a tech site somewhat underrated in our circles, had to review and correct dozens of AI-generated articles after significant errors were found in financial advice content. Some articles included incorrect compound interest calculations and misleading explanations of basic banking concepts that could have led readers to make harmful financial decisions.
Not to mention an Australian regional newspaper that published interviews partially generated by AI containing quotes never spoken by public officials, including a local mayor. Reader trust was severely damaged when it emerged that the political statements attributed to the mayor were completely fabricated and amateurish…
Finally, Bloomberg had to issue a swift correction after an AI-generated article contained incorrect information about interest rate decisions that briefly impacted financial markets. This incident showed how AI hallucinations can have direct economic consequences. Naturally, statements by some heads of state have taken things to a whole new level—who knows if their claims could also be called hallucinations?
Even the academic world, despite its emphasis on rigor and verification, is not immune to the pitfalls of AI hallucinations. Meta had to quickly withdraw its Galactica model in 2022 after researchers demonstrated that the system generated seemingly authoritative scientific papers containing fabricated methodologies, made-up results, and citations of nonexistent studies.
The scientific community has also documented worrying cases of students and even researchers using AI-generated content with fictitious references in their academic work. Some universities have found theses containing entire sections of completely hallucinated scientific literature, with plagiarism detection systems unable to flag this content as unoriginal because it is technically “original” — albeit false. A strange paradox: catching those who copy but not those who invent.
In the corporate world, AI hallucinations have caused both reputational and financial damage. The most glaring example is probably Google, which lost about $100 billion in market value during the launch of its Bard chatbot after the AI made a mistake about an astronomical discovery during a public demo.
Samsung, on the other hand, faced a security crisis when employees uploaded proprietary code to ChatGPT for programming assistance, only to discover that the AI subsequently incorporated snippets of this confidential code in responses to other users—potentially compromising valuable intellectual property. Again, nothing new here: years ago, I worked at a company that accidentally posted database credentials on Stack Overflow while seeking a solution to a problem—a mistake that cost months of headaches and thousands of euros.
Conclusion
The methods may change, but the human factor always plays the heaviest role.
Yet it’s precisely this human element that will solve the problem—by ensuring institutionalized oversight and prioritizing accurate content.
The alternative is blind trust in these “accepted” results, opening apocalyptic scenarios where honesty and credibility no longer matter, only the number of times a baseless “truth” appears statistically. In that case, whoever controls the past will have an easy game controlling the future.