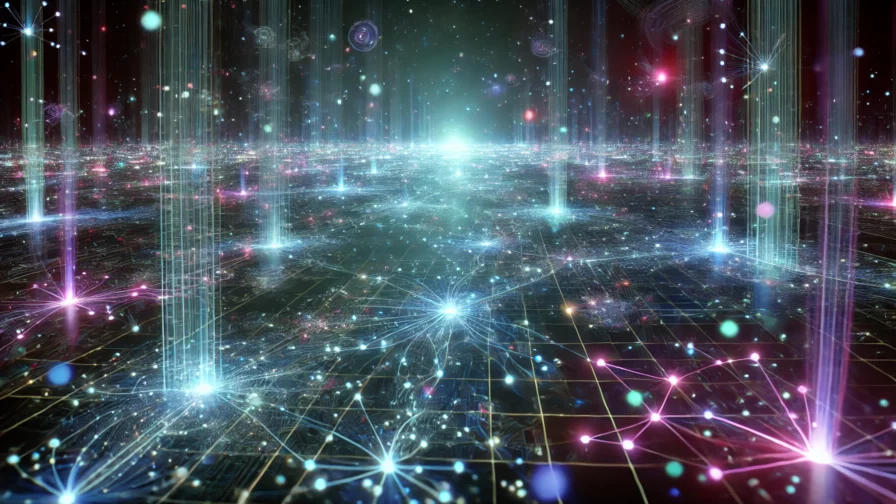
Insights from TeamSystem on Scalable, Adaptable, and Intelligent AI Systems
- 🔍 Abstract
- 1. The Enterprise AI Dilemma: Why Traditional Architectures Fall Short
- 2. Agentic Mesh Architecture: A Smarter Approach to AI Scalability
- 3. How TeamSystem is Building an AI Platform for Business Autonomy
- 4. Orchestrating AI Agents: The Challenges and Breakthroughs
- 5. The Future of Enterprise AI: What's Next for Scalable AI Architectures?
- Conclusion
🔍 Abstract
As artificial intelligence becomes a critical driver of business innovation, enterprises face a fundamental challenge: building scalable and adaptable AI architectures while avoiding rigid, monolithic models. Agentic Mesh Architecture is emerging as a solution, offering a framework where AI agents operate independently yet collaborate within a larger system.
TeamSystem is at the forefront of this evolution, developing an AI platform that allows different business units to create and manage their own AI agents in a low-code environment. This approach fosters flexibility, enabling AI to be tailored to different business needs without extensive development overhead. In this article, we explore how TeamSystem is applying this concept, the challenges of orchestrating AI agents, and what the future holds for enterprise AI architectures.
1. The Enterprise AI Dilemma: Why Traditional Architectures Fall Short
The story of enterprise AI implementations often follows a familiar pattern: A company makes a significant investment in a centralized AI solution, hoping it will transform operations across departments. Six months later, the marketing team complains the system doesn’t understand their specific needs, finance finds it lacks precision for their forecasting requirements, and customer service discovers it can’t handle the nuances of their interactions.
This scenario plays out in companies worldwide because traditional AI architectures weren’t designed for the complex, multi-faceted nature of modern enterprises. When organizations try to build one AI system to rule them all, they invariably create something that does many things adequately but nothing exceptionally well.
Beyond the performance issues, these centralized approaches create organizational bottlenecks. When every AI enhancement request must funnel through a single development team, innovation stalls. Business units with urgent needs find themselves in lengthy queues, watching as their market opportunities slip away while waiting for technical resources.
The reality is that a global financial services team needs AI capabilities fundamentally different from those required by a product development group or regional sales team. Forcing these diverse functions to use the same AI toolset isn’t just inefficient—it actively hampers business agility and innovation potential.
2. Agentic Mesh Architecture: A Smarter Approach to AI Scalability
Imagine instead an enterprise where each department has its own specialized AI agents, designed specifically for their domain challenges. The finance team works with agents that are good at numerical analysis and trend forecasting. Customer service deploys agents with deep natural language understanding and emotional intelligence. Marketing leverages agents with strong content generation capabilities and audience insights.
This is the promise of Agentic Mesh Architecture—specialization rather than generalization.
What makes this approach transformative is how these specialized agents work together. When a complex business process spans multiple departments, the agents collaborate seamlessly—each contributing its expertise while maintaining its domain focus. It’s like watching a well-orchestrated team where specialists handle different aspects of a project while staying aligned on the overall goal.
The business impact of this approach can be profound. When a specific team needs a new capability, they can enhance their dedicated agents without disrupting other operations. When regulatory requirements change for compliance, those specialized agents can be updated without slowing innovation elsewhere in the organization. The result is greater agility, faster innovation cycles, and better performance across all business functions.
3. How TeamSystem is Building an AI Platform for Business Autonomy
TeamSystem’s implementation of this vision feels surprisingly organic when you see it in action. They’ve structured their platform using an “onion architecture” that mirrors how humans naturally think about complex tasks.
At the outer layer are the agents themselves—AI entities with specific business purposes like invoice processing or customer communication. These agents aren’t single monolithic systems but compositions of multiple capabilities working in concert.
Moving inward, each agent orchestrates various tasks—discrete activities that deliver specific value. An invoice agent might read documents, extract key data points, validate compliance, or generate new invoices based on templates.
At the core sit the tools—the fundamental building blocks that enable each task. These might include database connectors, computational engines, or visualization components.
“The platform is built entirely on a proprietary framework, developed in Python, that guarantees us complete control and predictability over what the agents can execute,” they explain. “This foundation gives us the confidence to push boundaries while maintaining enterprise-grade reliability.”
What makes this approach original is how the responsibilities are divided. The technical team focuses on building robust, reliable tools and platform infrastructure. Meanwhile, business units—the people who truly understand their domains—configure agents through low-code interfaces to address their specific needs.
The result feels less like traditional software development and more like watching business experts craft their own digital assistants. A finance specialist might combine document analysis tools with regulatory validation capabilities to create an agent that streamlines compliance reporting. They don’t need to understand the underlying AI technology—just how to combine capabilities to solve business problems.
4. Orchestrating AI Agents: The Challenges and Breakthroughs
Of course, creating a harmonious ecosystem of AI agents isn’t without challenges. When TeamSystem first explored this architecture, they faced a fundamental question: how do you ensure dozens or even hundreds of specialized agents work together coherently without creating chaos?
Their solution centers around a sophisticated orchestration system that acts as the conductor for this AI orchestra. When a request comes in—whether from a user or another system—this orchestrator analyzes what’s needed and choreographs the appropriate response.
Sometimes this means engaging a single specialized agent. Other times, it requires coordinating multiple agents in carefully sequenced interactions. Throughout the process, the orchestrator maintains context, ensuring that each agent has the information it needs without overwhelming the system with redundant data exchanges.
What’s particularly innovative about TeamSystem’s implementation is their approach to performance monitoring. As they describe:
“For performance monitoring, we’ve created an internal evaluation system based on a mirror framework: each tool is decorated with a monitoring layer that collects specific metrics during execution. This allows us to build, in real-time, a reliable indicator of the agent’s overall performance, measuring the effectiveness of each task and the quality of the responses generated.”
This sophisticated monitoring doesn’t just support reliability—it enables continuous improvement. By understanding exactly how agents perform in production, TeamSystem can refine their capabilities over time, creating a virtuous cycle of enhancement based on real-world usage rather than theoretical optimization.
In practice, this means they can spot when an agent starts delivering less accurate results or becomes slower to respond. They can identify which specific tools or tasks are causing issues and address them precisely. It’s preventative maintenance for AI systems, catching problems before they impact business operations.
5. The Future of Enterprise AI: What’s Next for Scalable AI Architectures?
Looking ahead, this approach offers a glimpse into how enterprise technology will evolve. The days of monolithic applications are giving way to ecosystems of specialized capabilities that can be combined and reconfigured as business needs change.
The implications for organizations preparing for this future are significant. Technical teams will need to shift from building all-encompassing applications to creating platforms that empower business experts. Meanwhile, business units must develop the skills to effectively configure and manage AI agents without depending on central IT for every enhancement.
What’s perhaps most exciting about this approach is how it might reshape organizational structures themselves. As AI agents take on more specialized work, human experts can focus on higher-value activities that require creativity, judgment, and interpersonal skills. The boundary between human and AI work will become more fluid, with both collaborating in ways that leverage their respective strengths.
Conclusion
The Agentic Mesh Architecture represents a fundamental shift in how enterprises harness AI—moving from rigid, centralized systems to flexible networks of specialized agents. TeamSystem’s implementation proves this approach isn’t just theoretically superior; it delivers tangible business benefits today.
Organizations can build AI systems that address their unique challenges by embracing specialized excellence over generalized capabilities. This approach will find them better equipped to thrive in a business landscape where adaptability isn’t just an advantage—it’s a requirement for survival.
🚀 Explore more about TeamSystem’s AI innovations at www.teamsystem.com.