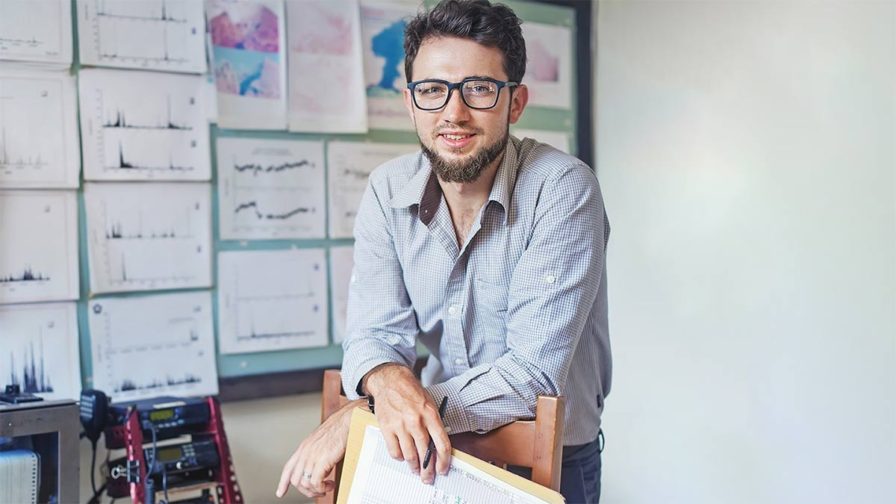
With every second we spend online, mountains of data is generated. For every social media post, Google search, and link clicked, there is a way in which our activity can be collected for data. Experts within the data science field can utilize this, creating meaningful information for businesses. Businesses can draw on this invaluable data to develop their customer base. This allows them to embrace new technologies and platforms – they might close sales using social media alone, or use AI to avoid cart abandonment. How? With the help of data analytics and data mining.
While both data mining and data analytics are a subset of Business Intelligence, that’s about all they have in common. One of the key differences between data analytics and data mining is that the latter is a step in the process of data analytics. Indeed, data analytics deals with every step in the process of a data-driven model, including data mining. Both fall under the umbrella of data science.
Data Science for Business Intelligence
For business owners, knowing their target audience’s behaviors and being able to capitalize on that information is like gold dust.
That’s where data science comes in. Specialists can provide genuine insight into a business’s customers – they can delve deeper and further than any traditional marketing method. This is because they can base their understanding on substantial evidence, rather than speculating about what the consumer may want. Data science uses extensive research to accurately forecast what steps a business may need to take in order to capture their audience and improve customer retention.
Both data mining and data analytics are needed to help a business strategize its next steps. Both demonstrate their own value to Business Intelligence, but what exactly are the key differences between them?
7 Differences Between Data Analytics and Data Mining
Team Size
Data mining can be undertaken by a single specialist with excellent technological skills. With the right software, they are able to collect the data ready for further analysis. At this stage, a larger team simply isn’t required. From here, a data mining specialist will usually report their findings to the client, leaving the next steps in someone else’s hands.
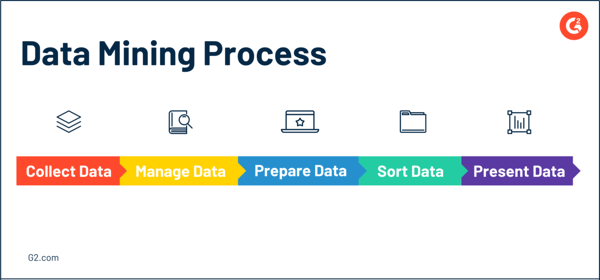
However, when it comes to data analytics, a team of specialists may be needed. They need to assess the data, figure out patterns, and draw conclusions. They may use machine learning or prognostication analytics to help with the processing, but this still has a human element involved.
Data analytics teams need to know the right questions to ask – for example, if they’re working for a telephony company, they may want to know the answer to ‘how is VoIP used in business’. A data mining specialist can provide evidence of where it’s used and how often, but data analytics uncovers the how and the why.
Their goal is to work together to uncover information and figure out how the gathered data can be used to answer questions and solve problems for the business.
Artificial Intelligence advances are likely to bring serious changes to the analytics process. An AI system can analyze hundreds of data sets and predict various outcomes, offering information about customer preferences, product development, and marketing avenues.
AI-powered systems will soon be able to complete menial tasks for data analytics teams, freeing up their time to take on more important work. It has the capability to dramatically improve the productivity of data scientists by helping to automate elements of the data analytics process.
Data Structure
When it comes to data mining, studies are conducted mostly on structured data. A specialist will use data analysis programs to research and mine data. They report their findings to the client through graphs and spreadsheets. This is often a very visual explanation, due to the complicated nature of the data. Clients are not typically data mining experts, and they don’t claim to be!
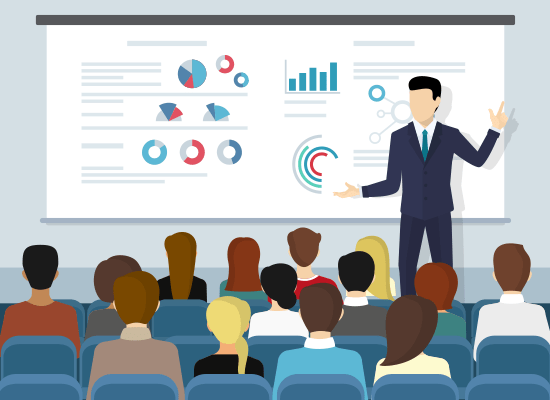
So, data needs to be fairly simply interpreted into graphics or bar charts. As with the earlier phone company example, if the client needs to know the data behind how many people click the link to ‘what is a VoIP number’ on their website, this should be displayed in easy to read charts, not complicated documents.
A data mining specialist builds algorithms to identify a structure within the data, which can then be interpreted. It’s based on mathematical and scientific concepts, making it excellent for businesses to gather clear and accurate data.
This is in contrast to data analytics, which can be done on structured, semi-structured, or unstructured data. They’re also not responsible for creating algorithms like a data mining specialist. Instead, they are tasked with spotting patterns within the data and using them to brief the client on their next steps.
This can then be applied to a company’s business model. The marketing team may want to see their customer and industry data laid out in front of them. If they can understand the behaviors of a competitor’s consumer, they can then apply it to their own strategies.
Data Quality
The way that the data needs to be presented for data mining compared to data analytics varies. While data mining is used to collect data and search for patterns, data analytics tests a hypothesis and translates findings into accessible information. This means the quality of data they work with can differ.
A dating mining specialist will use big data sets and extract the most useful data from them. Therefore, because they’re using vast and sometimes free data sets, the quality of the data they are working with isn’t always going to be top-notch. Their job is to mine the most useful data from this, and report their findings in ways businesses will understand.
However, data analytics involves collecting data and checking for data quality. Typically, a data analytics team member will be working with good quality raw data that is as clean as possible. When the data quality is poor, it can negatively impact the results, even if the process is the same as with clean data. This is a vital step in data analytics, so the team must check that the data quality is good enough to start with.
Hypothesis Testing in Data Analytics and Data Mining
A hypothesis is effectively a starting point that requires further investigation, like the idea that cloud-native databases are the way forward. The idea is constructed from limited evidence and then investigated further.
A key difference between data analytics and data mining is that data mining does not require any preconceived hypothesis or notions before tackling the data. It simply compiles it into useful formats. However, data analysis does need a hypothesis to test, as it is looking for answers to particular questions.
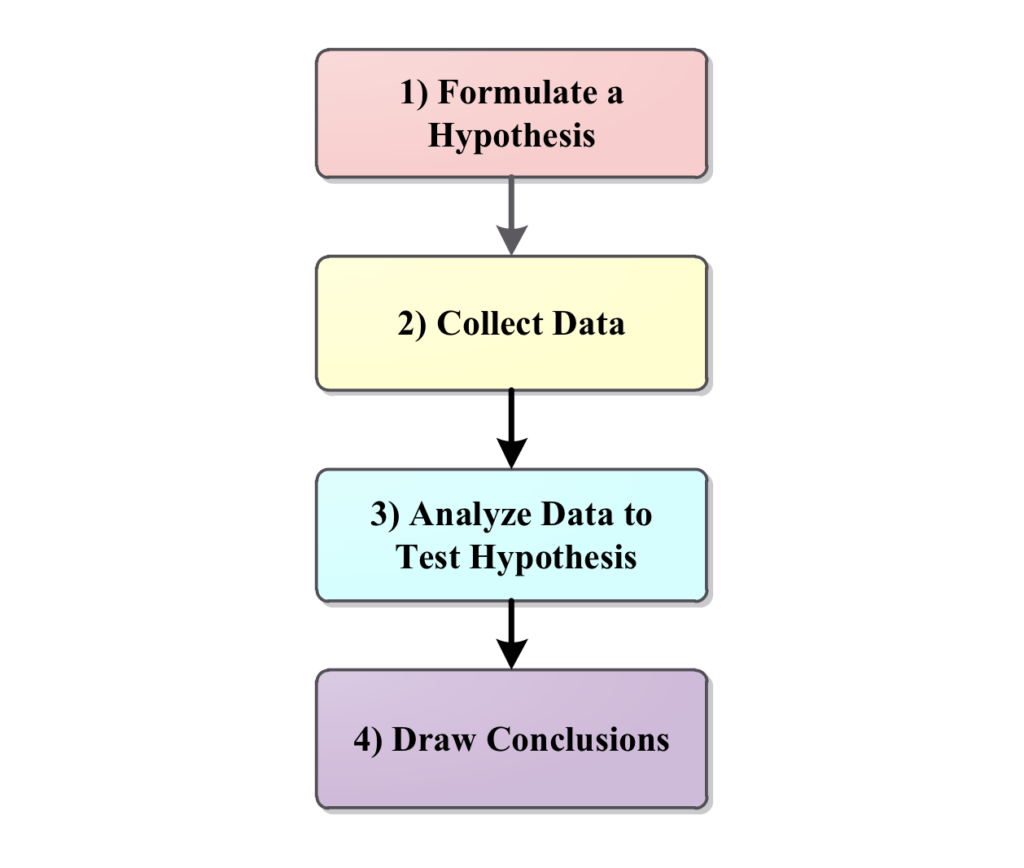
Data mining is about identifying and discovering patterns. A specialist will build a mathematical or statistical model based on what they derive from the data. Because they don’t lead with a hypothesis, a data mining specialist typically works with large data sets to cast the widest net of possibly useful data. This gives them the opportunity to whittle down the data, ensuring the data they’re left with at the end of the process is usable and reliable. This process works much like a funnel, starting with large data sets and filtering it into more valuable data.
In contrast, data analytics tests a hypothesis, extracting meaningful insights as part of their research. It helps in proving the hypothesis, and it may use the data mining discoveries in the process. For example, a business may start with a hypothesis such as, ‘Having a free sample link at checkout will lead to an improved conversion rate of 15%’. This can then be implemented and tested on the website.
The data analytics team will work to test the hypothesis statement by analysing each visit to the website. They may even conduct split tests into A/B for link placement, where ‘A’ leaves the sample link at the top of the page, and ‘B’ at the bottom. This gives an even closer insight into consumer behaviour when purchasing items, and lets the business know the best place to position the free sample link.
Forecasting
One of the tasks of a data mining specialist is forecasting what may be interpreted from the data. They find data patterns and note what it could lead to by using reasonable future predictions.
Understanding how the market may react to certain products or technologies can be important for brands and businesses across many sectors. Implementing a new technology such as a TCPA dialler brings both risks and benefits, and data can help a business decide if it’s the right solution for them.
Therefore, the work undertaken during the data mining process can prove to be essential for businesses that rely on forecasting trends.
As well as this, a data mining specialist will make sense of data by analyzing:
- Clustering – researching and recording groups of data, which is then analyzed based on similarities.
- Deviations – detecting anomalies within the data, and how and why this could have happened.
- Correlations – studying the closeness of two or more variables, determining how they are associated with one another.
- Classification – looking for new patterns in the data.
This all aids businesses in making smart decisions, based on genuine data from their consumers and the market they operate in.
On the other hand, data analytics is more about drawing conclusions from the data. It works partly in conjunction with data mining forecasts by helping to apply the techniques from its findings. Forecasting is not part of the data analytics process because it focuses more on the data at hand. They collect, manipulate and analyze the data. They can then prepare detailed reports drawing their own conclusions.
Forecasting is not to be confused with predictive analysis, which factors in a variety of inputs to then predict future behavior. It gives an overall view of past, present and future consumer behavior. So, it can also even be applied to events that have already happened.
Predictive analytics focuses more on statistics to predict outcomes. This could be beneficial for businesses who want to optimize marketing campaigns, though it does not give an insight into the market beyond this.
This differs from forecasting, which concentrates on predicting future trends in the market for years to come.
Responsibilities
The expectations of data analytics and data mining findings vary because both have different responsibilities.
While data mining is responsible for discovering and extracting patterns and structure within the data, data analytics develops models and tests the hypothesis using analytical methods.
Data mining specialists will work with three types of data: metadata, transactional, and non-operational. This is reflective of their responsibilities within the data analysis process. Transactional data is produced on a daily basis per ‘transaction’, hence the name. This includes data from customer clicks on a website. For instance, if you’re a software company, you may track how many customers click through from searches like ‘best UCaaS providers’.
Non-operational data refers to data produced by a sector that can be utilized to a company’s advantage. This involves investigating the data for insights and then forecasting for the future. What’s more, metadata refers to the database design, and how it holds the other data. This includes breaking the data down into categories, such as field names, length, type etc. Because it’s organized in this way, specialists find it easier to retrieve, interpret or use this information.
A data mining specialist’s responsibilities are often concerned with the way the data is collected and presented. Here’s an example of how metadata is used to organize information and present it:
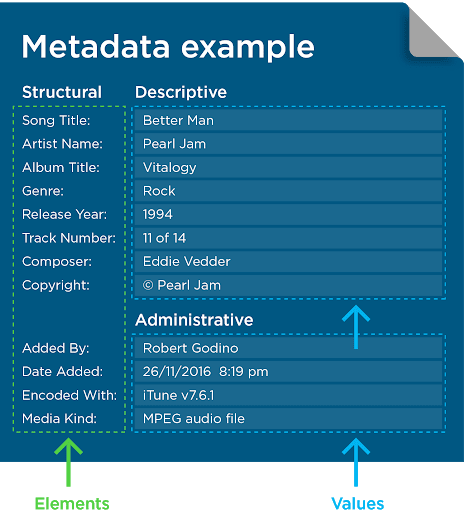
However, in data analytics, the team’s responsibilities are less about algorithms and more about interpretation. They predict yields and interpret the underlying frequency distribution for continuous data. This is so they can then report on relevant data when completing their tasks.
Companies usually look to data analytics teams to assist them in making important strategic decisions. This is one of their biggest responsibilities. Here are the different types of data the team may analyze:
- Social media content engagement and social network activity
- Customer feedback from emails, surveys and focus groups
- Page visits and internet clickstream data
The findings from these investigations can lead to new revenue opportunities as well as improved efficiency within the business. Their responsibility is to ensure that they produce consistent results that can be used as guidance for the future.
Area of Expertise
If you’re considering a career in data mining or data analytics, you need to be aware of the different areas of expertise required to take on the job.
Data mining is a combination of machine learning, statistics and databases. Data mining specialists need to master:
- Experience with operating systems such as LINUX
- Public-speaking skills
- Programming languages such as Javascript and Python
- Data analysis tools such as NoSQL and SAS
- Knowledge of industry trends
- Machine learning
This unique combination of technical, personal, and business skills is what makes a data mining specialist sought after within the industry.
Data analytics requires a different set of skills, namely in computer science, mathematics, machine learning, and statistics. Those who desire a data analytics career need to have:
- Strong industry knowledge
- Good communication skills
- Data analysis tools such as NoSQL and SAS, as well as machine learning
- Mathematical skills for numerical data processing
- Critical thinking skills
By using those with a skill set as described above, teams should be able to collect and analyze data, and provide a detailed report using project planning tools for the process. Putting together a team of people all with strong data analytics skills can take time, due to the specific requirements.
Using Data Analytics and Data Mining for Business Planning
We’ve looked at seven key differences between data analytics and data mining. Now it’s important to consider why both of these methods are needed for business planning.
A new business may look to data mining specialists and data analytics teams to gain further knowledge of the market they want to enter. This information can be used as part of their business plan, and may even help them secure investment. Unsurprisingly, a data-backed business plan is enticing to investors.
It can also help the business on a continuous basis. Just because data analysis can be used to forecast future trends, doesn’t mean data mining and data analytics should be used just the once. It can be useful for businesses to keep analyzing their data, particularly where there is a change in the economy or consumer habits. They can keep on top of ecommerce website maintenance using this information.
Well-established companies may also use data science to reinvigorate the brand. Analytics can help brands gain a better understanding of what their audience wants. This can be especially useful if a brand feels as though their presence has shifted, or if they’ve experienced a competitor become more successful than them.
A good example of this is fax machines. Before email, fax machines were relevant and at the top of their game. Since the rise of the internet, people use them far less. Companies with strong data science may have gotten ahead of the trend, focusing on how to fax from a computer rather than dedicated machines. This enables them to remain relevant, and develop to suit the current market
While there are many differences between data analytics and data mining, businesses should utilize both if they want a thorough understanding of how they can improve their brand and generate better engagement from consumers.