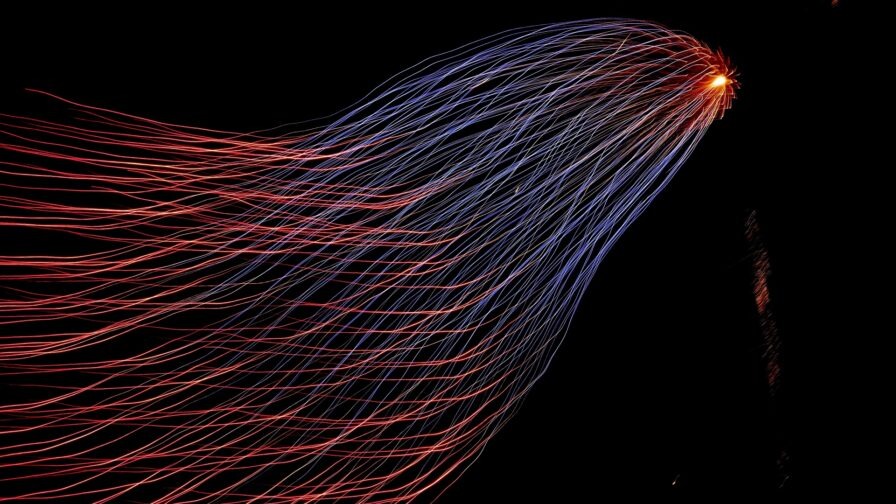
Do you remember all those headlines declaring “Data Scientist” as the hottest job of the century? Well, that was a decade ago. So, what has happened since then? Let’s take a look at some of the latest data science trends.
- Google is running a professional certificate course in data analytics to bridge the talent gap in the industry.
- Even today, it takes businesses 280 days on average days to identify and limit a data breach.
- Natural Language Processing has gone mainstream.
While these might appear to be primary topics of discussion in data science for 2022, they are mere offshoots of undercurrents with much more impact. From security to efficiency to making data science more accessible, here are the most mind-bending data science trends you should know about in 2022.
1. Edge Intelligence
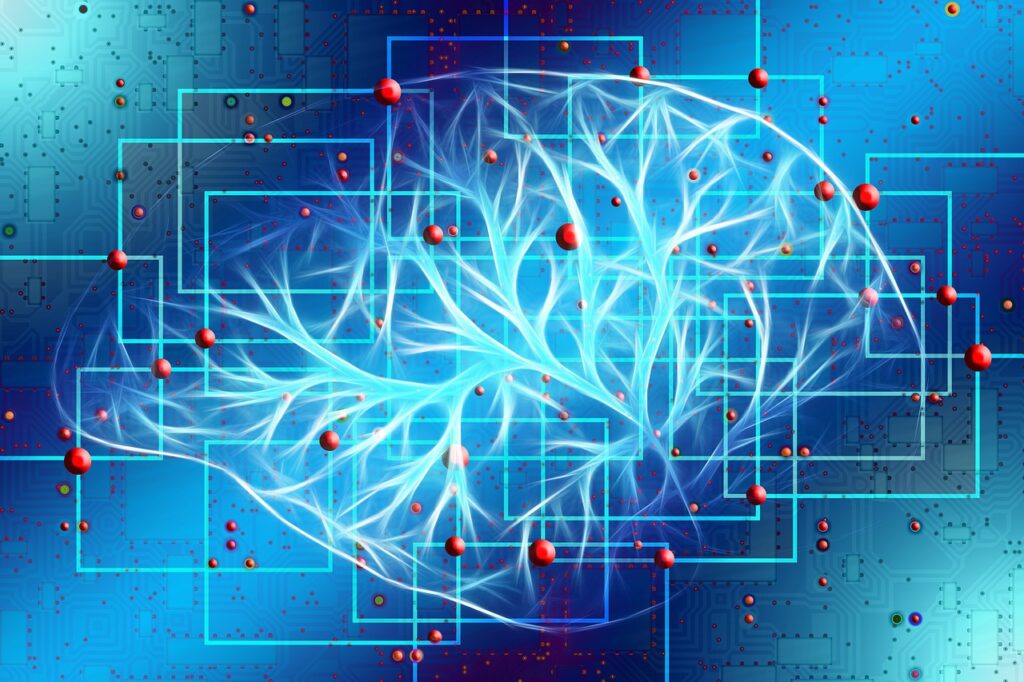
Cloud computing has been touted as the future of scalable AI. However, training and deploying AI models in the cloud has its wide range of challenges:
Wide Area Networks (WANs): While the data is stored and processed in the cloud, the transmission process requires WAN. The legacy infrastructure can often create cost barriers at entry and scale.
Latency Issues: Full accessibility in the cloud depends on the precise functioning of every touchpoint across the network. However, maintaining this level of performance for extended periods is impossible; hence, there are latency or downtime issues even with cloud systems.
Privacy Challenges: Even with a long history of data stored and processed in the cloud, despite enterprise-grade security, there will be cybersecurity risks. As a matter of fact, by 2025, as much as 80% of companies with suboptimal data governance practices will not be able to scale their digital business.
Imagine a system where the data is processed and used exactly where it is collected. This can be an IoT device or an endpoint device that is interacting directly with the user who is generating the data. By simply shifting the responsibility of collecting, processing, and analyzing the data at the “edge” instead of the cloud, three major challenges – suboptimal economics, latency issues, and privacy challenges can be solved. This is what edge computing is all about.
Applications such as CCTV monitoring and live video streaming are executed to their full capacity at the device level instead of data being sent back to the cloud or a data center to be processed and analyzed.
Developers and companies choose between different combinations of cloud and edge to train the AI models, process data, and deliver insights. As AI systems become more efficient at scale, edge data collection, processing, and analysis becomes more accessible.
A layer above edge computing is the new area of research and application called edge intelligence. If we are storing and accessing data at the edge, we should analyze it with AI models at the edge, right? While it is still relatively unexplored territory, the answer seems to be Yes.
2. Observability
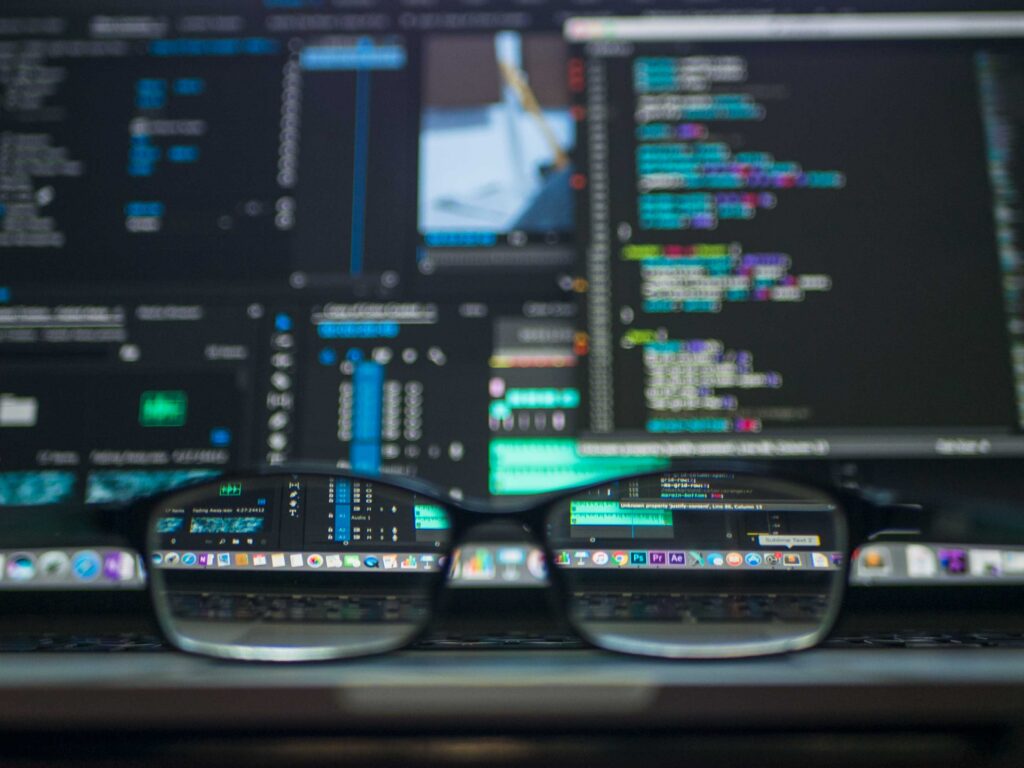
If you intuitively understand metrics, tracking and monitoring, you can graduate to the idea of observability, which revolves around complex systems where self-adaptive elements and asymmetric consequences (chaotic behavior) are common.
When you proactively collect, visualize, and apply intelligence to metrics and logs within this complex system, you can understand its behavior better. Therefore, a simple way of defining observability is understanding an IT system by observing the work it does.
Now, try to observe the modern software development lifecycle with Kubernetes, distributed development teams, and continuous integration and continuous delivery (CI/CD). It becomes very easy to lose grip on all the moving parts and establish the cause of the errors.
Monitoring is somewhat diagnostic because you understand specific indicators as the causal factors for failure. Hence, as far as you can correlate these factors across a timeline, you can diagnose the issue at hand before it scales. Observability goes beyond this idea and includes metrics, events, logs, and traces to deliver a more comprehensive picture.
In terms of process, observability depends on telemetry data collection and absolute visibility across the topography of critical assets in the network.
Moreover, the data collected must be backed by metadata (data about data, or attributes of data) that helps establish the proper context for further analysis. Companies that can use metadata are projected to increase their data team productivity by as much as 20%. With such contextual intelligence available, it would be easier to automate a substantial amount of IT operations and workflows intelligently.
3. Customer Analytics
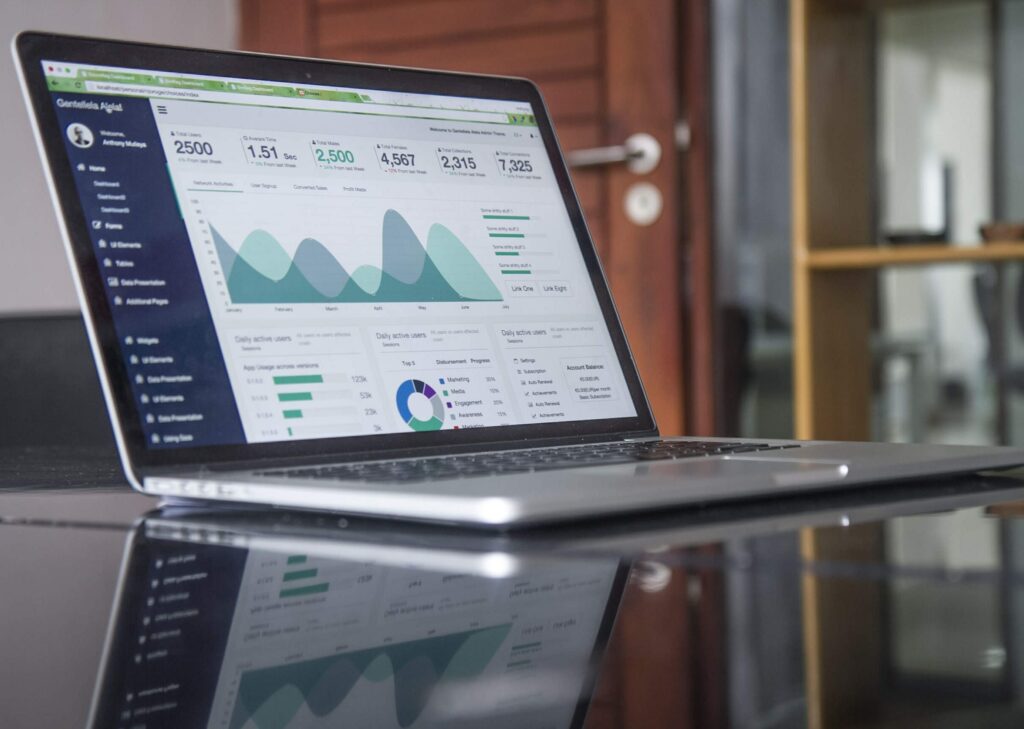
Data has been at the core of several successful products and companies. But, with more and more granular data available on user actions and behavior online, the playing field has been leveled for small businesses and startups to leverage accessible insights at par with enterprises.
Customer analytics can be a broad term used to assess user behavior on a platform. Since technology products are easier to track, they lend themselves to many service-oriented applications.
The most popular and universal use today is in automation-focused CRM tools featuring web- or app-based chatbots that utilize deep learning models. These bots aim to understand the context of support tickets or platform-specific messages and recommend an appropriate course of action to customers. The system also learns from the outcome of each customer interaction and builds a knowledge base for marketing, sales, and customer service.
When implemented at scale, customer analytics can help in:
- Defining the latent needs of audiences for UI/UX optimization and feature engineering
- Developing similar user personas to streamline customer acquisition
- Predicting user behavior to nudge customers along the sales funnel and enable sales
- Assessment and forecasting of critical points of friction in the user journey
Customer analytics can make the user journey more seamless – from brand awareness to conversion to customer acquisition to brand advocacy. Businesses today have the option of a wide range of third-party analytics platforms like Mixpanel and Google Analytics to develop user analytics capabilities from the ground-up.
4. Hybrid Cloud Automation
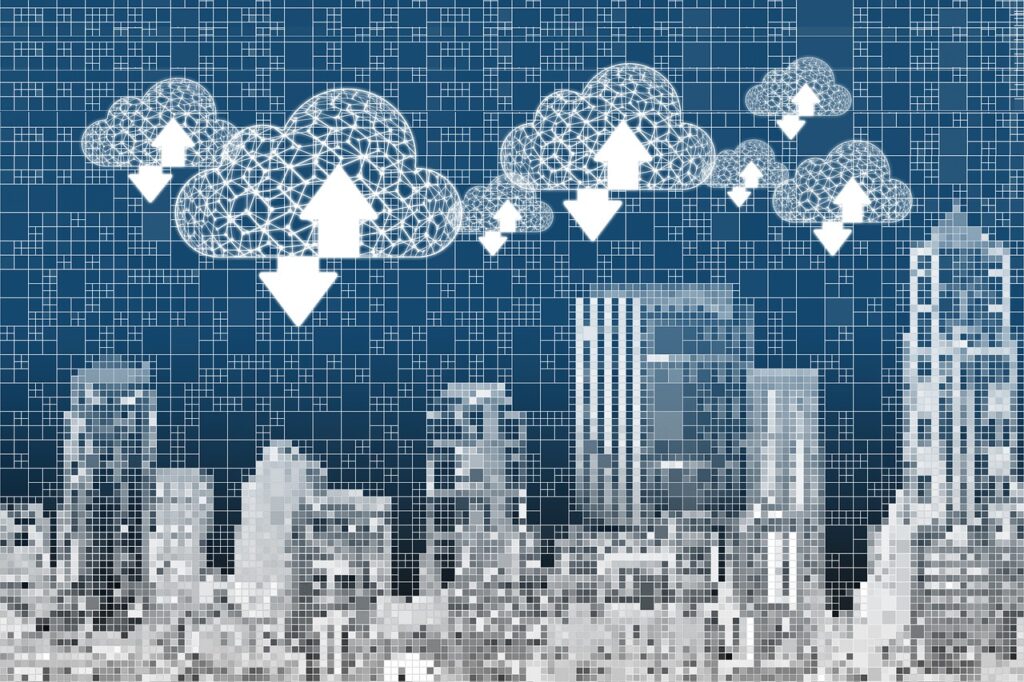
A hybrid architecture has been the antithesis to the idea of moving everything to the public cloud and having no infrastructure management in-house. Every application or workflow cannot be entirely cloud-based in an enterprise setup. Companies that want to maintain uptime, comply to data privacy regulations, and performance standards prefer a hybrid cloud architecture.
In fact, 86% of over 3,000 global IT leaders surveyed in the Enterprise Cloud Index report said that the hybrid cloud was their ideal operating model.
And this is where the management processes start getting tricky. Cloud architects are often subjected to challenges like aggregating data, designing and executing cloud balancing protocols, assigning & resolving IP addresses to machines, maintaining configuration management databases, and engineering the orchestration process.
Hybrid cloud automation makes it easier for cloud and network architects to automate most of these processes. Even a small degree of automation based on the inherent requirements of the business can lead to:
- Optimal utilization of hybrid cloud resources
- Ensure critical application uptime and accessibility
- Meet the pre-determined network uptime and performance goals
- Higher productivity of cloud architects and network administrators who can focus on strategic issues instead of firefighting
5. Hyperautomation
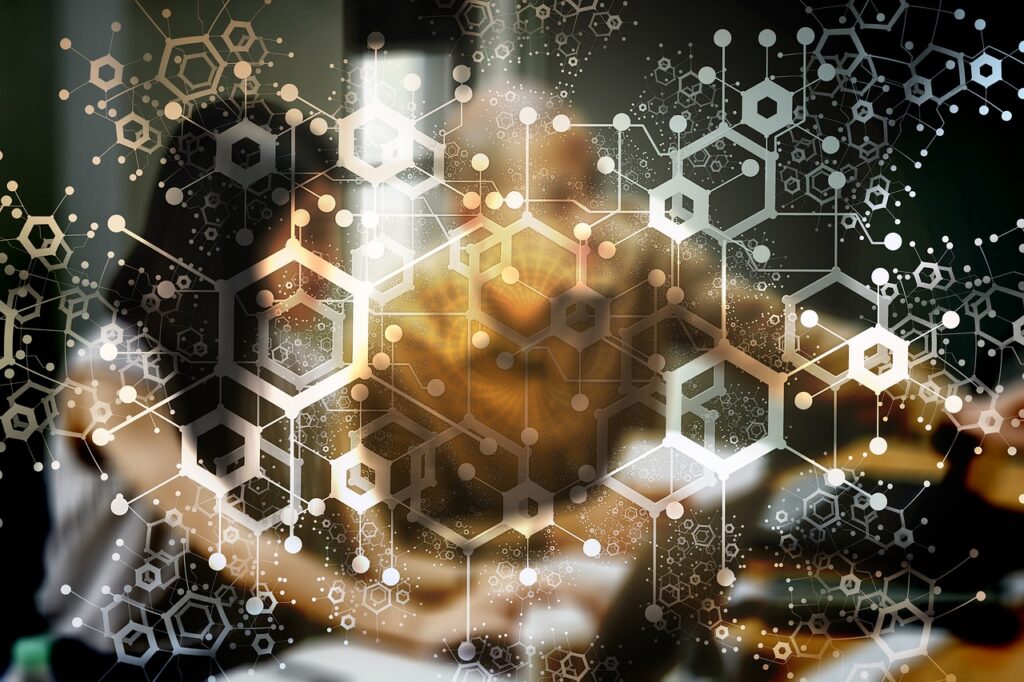
Most trends we’ve examined so far involve processes, systems, and technologies. Hyperautomation is a working “philosophy” evangelized by technology leaders like IBM and Gartner.
The idea of hyperautomation builds on our current understanding of automation. In a simplistic form, automation is a rule-based mechanism to transform a set of manual tasks into an automatic process. But, in the context of technology, this can be achieved in a bunch of ways:
- Artificial Intelligence (AI) and Machine Learning (ML) algorithms are trained and deployed at scale.
- Robotic Process Automation fits well in the enterprise context.
- Low Code or No Code tools enable citizen developers to create simple rule-based programs for automating daily tasks.
- Business Process Management (BPM) platforms offer automation capabilities after specific baseline data has been collected, vetted, and processed.
Hyperautomation focuses on effectively and efficiently automating every workflow or process in a business that can be automated. In that sense, it is an orchestration mechanism to select the tools, platforms, and technologies for transforming business processes on a continuum towards automation and enabling digital transformation in businesses of all sizes.
6. Democratizing AI
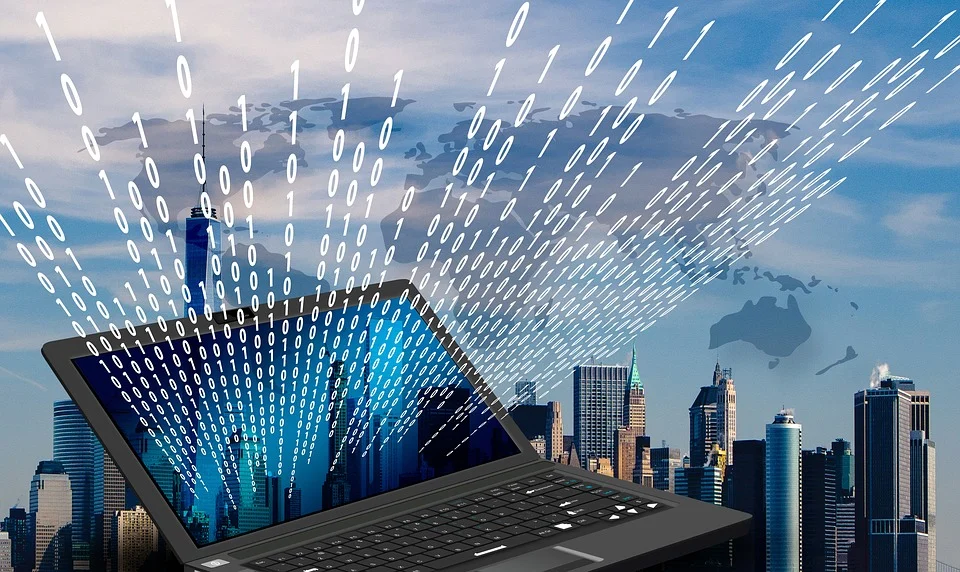
What the drag-and-drop movement did to web development, what the plug-and-play concept did to OS and hardware, the idea of democratizing AI does for data, algorithms, and entire marketplaces. AI democratization has become a hotly debated data science theme.
Tech giants such as Amazon, Google, and Microsoft are looking to make artificial intelligence accessible to anyone and give them the tools to build machine learning models without any coding knowledge, armed with just access to the internet and an essential computing device.
Why is democratizing AI even necessary? One, AI experts are few and far between. But the problems they are required to solve are virtually limitless. Since all businesses (especially startups or small businesses) can’t recruit trained data scientists with PhDs for each of their industry challenges, democratized AI solutions can make it easier for data analysts and senior executives to leverage the full capability of AI and ML, without having to depend on a team of data scientists.
Two, project owners and data scientists can work in tandem – the management team can demonstrate preliminary versions of the end product to data scientists, enabling them to create immediately-deployable, multi-functional digital products.
Onward and Forward
As more talented and knowledgeable data scientists enter the world of data science, they are helping uplift the quality of life for human beings. The data and analytics space is in for some major shakeups in 2022 and beyond. Businesses looking to improve brand experience for their customers would do well to monitor and leverage these trends if they want to have a go at delivering better business value and surge ahead of the competition. This is an ever-evolving field, so be always ready to discover and explore new data science trends!
Discover more about Data Science in this Codemotion video with Thiago de Faria
Languages for data science
As we explored in this post, data science is a rapidly growing field and one of the most important skills for developers to master. With so many languages and tools available, it can be daunting to figure out which language you should use for your projects. So let’s take a look at some of the most popular languages used in data science:
- Python is a top-choice programming language for those interested in data science. The vast community of users and developers has fostered a diverse ecosystem, brimming with libraries and frameworks like NumPy, Pandas, Scikit-Learn, TensorFlow, and PyTorch. Within this ecosystem, users are equipped to easily and effectively manipulate data, visualize it, and launch machine learning experiments. Even better, Python’s syntax is beginner-friendly and easy to read, making it an excellent fit for newcomers and programming veterans alike.
- R: Seeking a programming language that’s a statistical powerhouse for data visualization and manipulation? Look no further than R. This popular tool offers a thriving bundle of packages, like dplyr, ggplot2, and caret, that fortify your skills with data analysis, making it easier to master machine learning. R’s abilities for statistical computing are lauded by researchers and academics alike, fitting smoothly into research environments.
- Julia, the new kid on the block of programming languages, has taken the data science world by storm. Its secret weapons? Amazing performance and ease of use. Julia is built to tackle heavy-duty tasks like machine learning and numerical simulations with a lightning-fast response time. What’s more, it boasts a syntax that will make fans of MATLAB feel right at home. It’s no wonder Julia is quickly becoming the go-to choice for data scientists and engineers everywhere.
- Java is a versatile and widely used programming language that is also used in data science, particularly in big data and distributed computing environments. Java has a large ecosystem of libraries and frameworks for machine learning and big data processing, such as Apache Spark and Hadoop. Java’s strong typing and object-oriented features make it suitable for large-scale data processing and production-ready applications.
- SQL is a specific language designed for managing and searching through relational databases with ease. It is considered the backbone of data science, as it allows individuals to extract, alter, and analyze massive amounts of data with minimal effort. With SQL, users can quickly clean up and arrange data, gather information from multiple tables, and conduct detailed data analysis. It’s no wonder why data scientists rely on SQL to turn raw data into valuable insights.